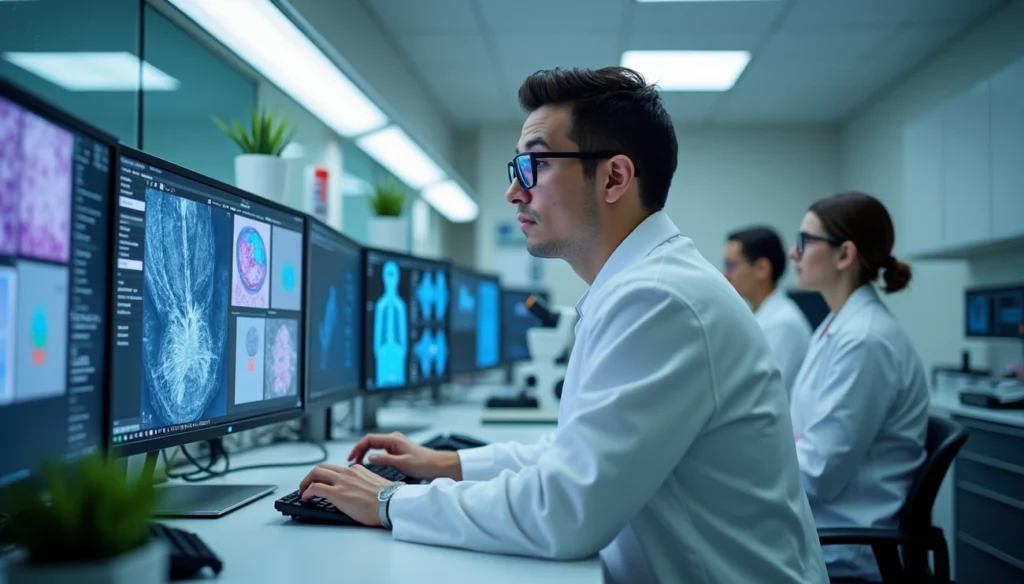
Artificial Intelligence in Diagnostics: Friend or Foe for Techs?
AI is changing how we diagnose and treat patients faster than ever before. Our world will face a shortage of 18 million healthcare professionals by 2030, including 5 million fewer doctors than needed. Medical errors cause about 100,000 deaths every year in the United States. These numbers show we just need groundbreaking solutions right now.
AI medical diagnosis brings new possibilities and challenges to diagnostic professionals. Healthcare diagnostics with AI will grow at 37.3% yearly from 2023 to 2030. This is a big deal as it means that AI medical diagnosis systems can now match or surpass what human experts can do in several specialties. These technologies are changing radiology and pathology, where we’ll need 26% more specialists between 2023 and 2055.
This piece looks at how AI is altering the map of diagnostics. We’ll get into the opportunities for healthcare professionals and tackle real concerns that diagnostic techs face. Let’s see how we can get ready for a future where AI increases our healthcare capabilities.
How AI is changing the diagnostic landscape
AI is radically changing traditional medical processes in the diagnostic world. This technology doesn’t just enhance existing methods – it creates new ways for healthcare professionals to detect and treat diseases.
From manual to machine: a change in workflow
Medical teams traditionally start with physician examinations and then gather data for assessment. Recent studies show AI has completely reversed this process. Medical teams in advanced settings now receive AI recommendations from imaging data right at the start [1]. Medical professionals have adapted by creating new approaches that start with AI suggestions. They work backward through multiple verification steps to maintain their vital role while using AI’s capabilities [1].
AI in medical imaging and pathology
Medical imaging represents AI’s biggest impact in diagnostics. Healthcare facilities conduct about 3.6 billion imaging procedures each year, and 97% of this data went unused historically [2]. The radiology field alone now has nearly 400 FDA-approved AI algorithms [2]. AI systems achieved 90% sensitivity in breast cancer detection compared to radiologists at 78% [3]. Deep learning algorithms spotted pneumonia in chest radiographs with 96% sensitivity while human readers achieved 50% [3].
Rise of AI in healthcare diagnostics
AI continues to grow rapidly in healthcare. Only 25% of healthcare organizations use AI now, but 91% believe it will drive their success within five years [4]. These algorithms excel at finding anomalies in medical images, spotting trends in patient data, and connecting symptoms to possible conditions [2]. AI-powered tools help radiologists find early-stage cancers in lung nodule CT scans that might slip past human eyes [2]. On top of that, it helps stroke teams by instantly sharing MRI/CT images on mobile devices and identifying vital markers like large vessel occlusions [1].
Opportunities AI brings to diagnostic professionals
AI helps diagnostic professionals who face burnout in their daily work. Nearly half of U.S. physicians report burnout symptoms, mainly due to administrative overload and repetitive tasks [5]. AI technologies create new opportunities that could transform their work experience and patient outcomes.
Reducing repetitive tasks
AI systems excel at handling time-consuming, high-volume repetitive tasks, especially in precision imaging [6]. Lab automation through AI and robotics lets machines handle sample preparation and analysis, which reduces human workload [7]. Automated documentation tools save up to two hours per provider each day. This allows clinicians to focus on patient interaction rather than paperwork [5]. AI-powered robotic systems have achieved a 50% reduction in sample processing time in clinical laboratories while maintaining 99.5% accuracy in sample preparation [8].
Improving diagnostic accuracy
AI’s effect on diagnostic precision is remarkable. The average human diagnostic error rate in the U.S. is approximately 11.1%, with nearly 98,000 annual deaths linked to medical mistakes [9]. AI algorithms have shown remarkable results:
- AI detected breast cancer with 90% sensitivity compared to 78% for radiologists [3]
- Deep learning algorithms identified pneumonia from chest radiographs with 96% sensitivity versus 50% for human readers [3]
- AI diagnosed cancer risk from mammograms 30 times faster than physicians with 99% accuracy [9]
ChatGPT scored a median of 92% (equivalent to an ‘A’ grade) in diagnostic reasoning tests, performing better than physicians who scored around 75% [10].
Faster turnaround times for test results
AI speeds up diagnostic processes effectively. Clinical laboratories that use AI-powered systems see a 30% reduction in processing errors and a 40% increase in throughput [8]. AI reduces time-to-diagnosis for certain diseases by 30%, which enables quicker treatment initiation [8]. AI tools analyze vast quantities of imaging data that would otherwise go unused (97% of 3.6 billion annual imaging procedures) in radiology [11]. This improved efficiency leads to better patient outcomes and reduces healthcare costs through fewer hospitalizations and precise resource use [12].
Challenges and concerns for diagnostic techs
AI offers huge benefits to diagnostic professionals, but its integration into healthcare workflows faces major challenges. Healthcare professionals must think over these concerns to adopt these powerful tools successfully.
Job displacement fears
Job security stands as a top concern for diagnostic technicians. A 2024 Gallup poll showed that nearly 25% of workers worry AI could make their jobs obsolete, up from 15% in 2021 [13]. Chief human resources officers’ predictions show over 70% believe AI will replace jobs within three years [13]. The World Economic Forum projects automation will displace 85 million jobs by 2025 [13]. Healthcare workers voice specific concerns about job obsolescence, expertise devaluation, and uncertain career futures [14].
Skill gaps and training needs
The AI skills gap poses another significant challenge. Pathologists maintain positive attitudes toward AI, but many lack knowledge about its practical use [15]. Business leaders estimate 40% of their workforce needs new skills over three years [13]. Notwithstanding that, organizations find it hard to scale training programs while AI technology evolves faster. They struggle to optimize operations while keeping employees’ skills current [4]. Half of the organizations investing in reskilling face sustainability challenges due to cost and time barriers [4].
Overreliance on AI systems
Automation bias can result from over-dependence on AI systems [16]. Healthcare professionals’ growing reliance on AI recommendations raises real concerns about declining clinical skills [17]. This dependence might affect doctors’ ability to make independent decisions when AI systems fail or become unavailable [17]. Patient-healthcare worker relationships might also suffer as human interactions become less common [17].
Ethical concerns in AI medical diagnosis
Ethical issues spread through every aspect of AI in diagnostics. Algorithmic bias creates significant challenges that lead to diagnosis disparities among patient groups [18]. Patient data protection raises privacy concerns [18]. The question of accountability remains unresolved – determining responsibility becomes complex when AI systems make mistakes [19]. These ethical aspects need careful consideration to ensure AI boosts rather than undermines patient care.
Preparing for an AI-augmented future
Healthcare professionals must adapt strategically to what a world of AI and human expertise joining together looks like. Most fields now see professional skills becoming outdated in less than five years [20]. Diagnostic technicians should embrace new approaches to stay relevant in an AI-enhanced healthcare system.
Upskilling and continuous learning
Healthcare professionals who understand AI are in short supply. The global workforce will just need reskilling by 2030 [20], with more than one-third of C-suite leaders seeing AI as their top training investment [21]. Healthcare organizations are taking steps to close this gap:
- AI analyzes individual strengths and knowledge gaps to create customized training plans [22]
- Virtual simulations let professionals practice with AI-powered patient scenarios on demand [22]
- Feedback systems assess clinical performance and offer instant improvement suggestions [22]
Organizations that invest in AI-driven upskilling see attrition rates four times lower among participating employees [20]. This makes continuous learning both necessary and advantageous.
Collaborating with AI tools
AI tools work best when professionals see them as partners rather than replacements. Healthcare outcomes improve significantly when human expertise combines with AI capabilities. Dermatologists achieve better results in diagnosing skin conditions among other AI tools [12]. This collaborative effort should emphasize:
- AI acts as another set of eyes to flag potential issues that physicians can investigate [23]
- AI handles routine tasks while professionals tackle complex decisions [23]
- Health reports blend AI analysis with patient data [23]
Shaping policies for responsible AI use
Healthcare organizations must build governance frameworks based on core principles to ensure ethical AI use. An all-encompassing approach has nine key principles: fairness, equity, robustness, privacy, safety, transparency, explainability, accountability, and benefit [24]. Therefore, effective governance requires:
Teams with experts from different fields including data scientists, clinicians, ethicists, and social scientists [2] Systems that monitor and assess AI performance in different demographics [24] Standard protocols for collecting data and developing algorithms [2]
These frameworks ensure AI remains a tool that amplifies human intelligence without replacing the essential human aspects of medical care [6].
Conclusion
Without doubt, AI in medical diagnostics serves as both an ally and potential threat to diagnostic professionals. Our exploration reveals AI systems that match or exceed human accuracy across specialties and reduce repetitive task burdens. In spite of that, we cannot ignore valid concerns about job displacement, skill gaps, and ethical implications.
The future of diagnostics belongs to adaptable professionals rather than resistors. Medical professionals who want to succeed in this changing environment must prioritize skill development. Research demonstrates that collaborative efforts between humans and AI yield better results than either working independently. We should call it a powerful tool that handles routine tasks while medical professionals focus on complex cases that need human judgment.
The transition might feel overwhelming, but embracing continuous learning will lead to improved patient outcomes and more rewarding careers. Healthcare professionals must help shape ethical frameworks that govern AI use. AI systems reflect the quality of humans who design, implement, and work with them. AI has already reshaped the diagnostic scene. The real question focuses on our adaptation strategies to ensure this change benefits healthcare professionals and patients alike.
FAQs
Q1. How is AI changing the field of medical diagnostics? AI is transforming medical diagnostics by automating repetitive tasks, improving diagnostic accuracy, and speeding up test result turnaround times. It’s particularly impactful in medical imaging and pathology, where AI algorithms can detect anomalies and patterns that might be missed by human eyes.
Q2. What opportunities does AI bring to diagnostic professionals? AI offers several opportunities for diagnostic professionals, including reduced workload on repetitive tasks, improved diagnostic accuracy, and faster processing of test results. This allows healthcare workers to focus more on complex cases and patient interaction, potentially reducing burnout and improving job satisfaction.
Q3. What are the main concerns for diagnostic technicians regarding AI implementation? The primary concerns include fears of job displacement, the need for new skills and training, potential overreliance on AI systems, and ethical issues related to AI in medical diagnosis. There’s also worry about maintaining clinical skills and the impact on patient-healthcare worker relationships.
Q4. How can diagnostic professionals prepare for an AI-augmented future in healthcare? Diagnostic professionals can prepare by embracing continuous learning and upskilling, particularly in AI-related areas. They should also focus on developing skills that complement AI capabilities and actively participate in shaping policies for responsible AI use in healthcare.
Q5. Is AI more accurate than human diagnosticians? In some areas, AI has demonstrated higher accuracy rates than human diagnosticians. For example, AI has shown superior performance in detecting certain cancers from medical images and identifying pneumonia from chest radiographs. However, the most effective approach appears to be a collaboration between AI and human expertise, combining the strengths of both.
Disclaimer: The viewpoint expressed in this article is the opinion of the author and is not necessarily the viewpoint of the owners or employees at Healthcare Staffing Innovations, LLC.
References
[1] – https://www.nature.com/articles/s41746-025-01460-1[2] – https://www.cdc.gov/pcd/issues/2024/24_0245.htm
[3] – https://bmcmededuc.biomedcentral.com/articles/10.1186/s12909-023-04698-z
[4] – https://www.newhorizons.com/resources/blog/ai-talent-gap
[5] – https://medcitynews.com/2024/11/reducing-clinical-and-staff-burnout-with-ai-automation/
[6] – https://pmc.ncbi.nlm.nih.gov/articles/PMC8285156/
[7] – https://www.weforum.org/stories/2024/05/how-ai-robotics-and-automation-will-reshape-the-diagnostic-lab-of-the-future/
[8] – https://blog.creliohealth.com/the-ai-revolution-in-clinical-laboratories-shaping-future-of-diagnostics/
[9] – https://www.forbes.com/councils/forbesbusinesscouncil/2025/03/06/accelerating-healthcare-with-ai-improving-diagnostic-accuracy/
[10] – https://hai.stanford.edu/news/can-ai-improve-medical-diagnostic-accuracy
[11] – https://www.aha.org/aha-center-health-innovation-market-scan/2023-05-09-how-ai-improving-diagnostics-decision-making-and-care
[12] – https://www.mgma.com/articles/artificial-intelligence-in-diagnosing-medical-conditions-and-impact-on-healthcare
[13] – https://www.ibm.com/think/insights/ai-upskilling
[14] – https://pmc.ncbi.nlm.nih.gov/articles/PMC11003342/
[15] – https://pubmed.ncbi.nlm.nih.gov/39542175/
[16] – https://pmc.ncbi.nlm.nih.gov/articles/PMC7973477/
[17] – https://pmc.ncbi.nlm.nih.gov/articles/PMC11344516/
[18] – https://pmc.ncbi.nlm.nih.gov/articles/PMC11249277/
[19] – https://pmc.ncbi.nlm.nih.gov/articles/PMC11612599/
[20] – https://amplyfi.com/blog/ai-driven-skill-shift-the-need-for-continuous-upskilling/
[21] – https://www.bal.com/perspectives/six-winning-strategies-to-upskill-your-workforce-for-ai/
[22] – https://shccares.com/blog/workforce-solutions/healthcare-upskilling-with-ai/
[23] – https://www.smartbloodanalytics.com/en/waiting-room/collaborative-intelligence-how-ai-and-physicians-can-work-together-for-better-healthcare-outcomes-2354956
[24] – https://pmc.ncbi.nlm.nih.gov/articles/PMC11608363/